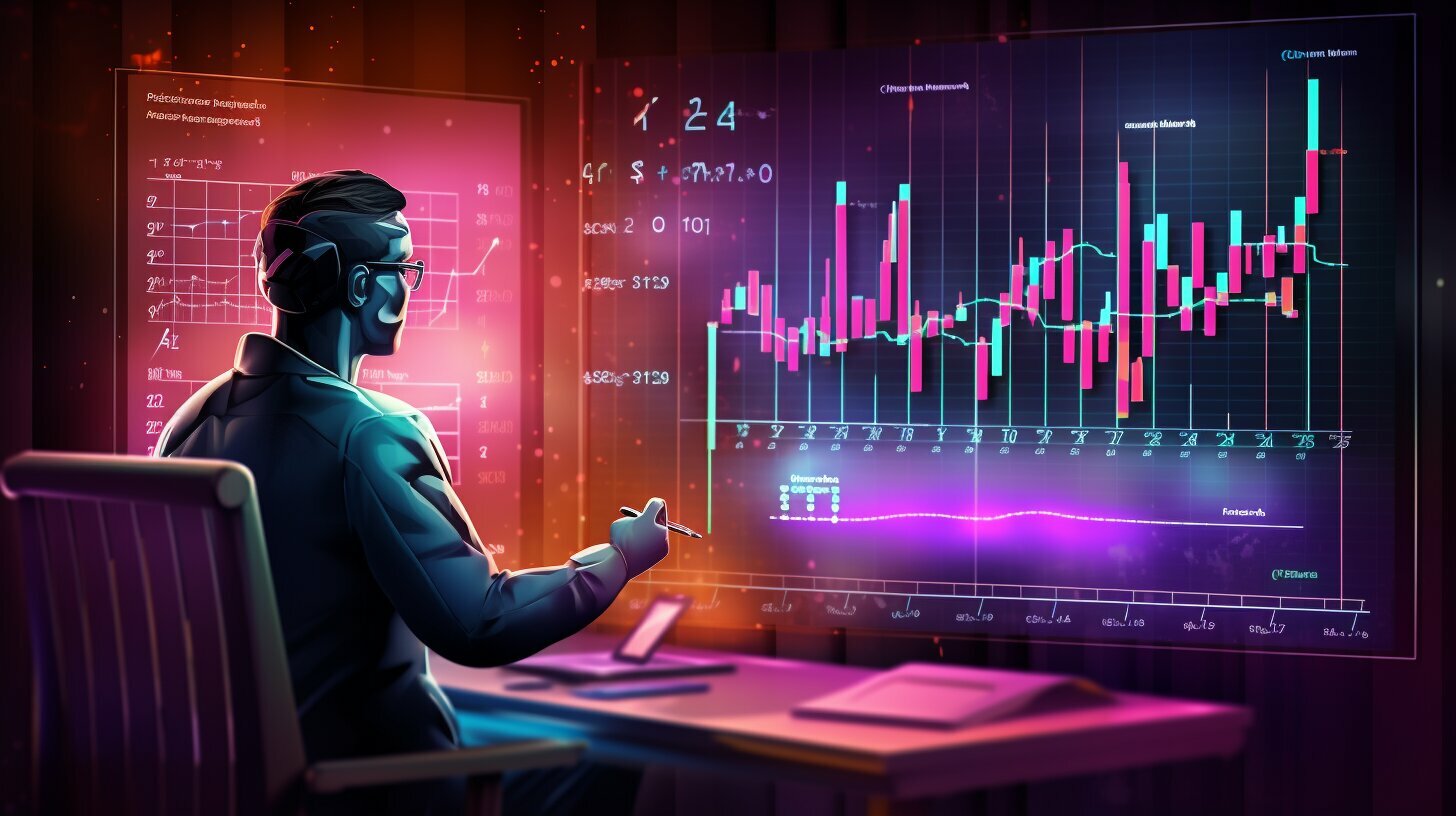
How to Utilize Machine Learning for Better Results in Lead Generation in the AI Era
In today's AI-driven business landscape, effectively utilizing machine learning strategies can revolutionize lead generation and yield better results. Industries across the board are embracing the power of machine learning to enhance their lead generation efforts and drive growth in the AI era. By harnessing the capabilities of machine learning algorithms and predictive analytics, businesses can uncover valuable insights, optimize their processes, and ultimately attract and convert more high-quality leads.
As technology continues to evolve, machine learning models have also seen significant advancements. From simple rule-based models to sophisticated deep learning networks, the evolution of machine learning has paved the way for improved lead generation strategies. Companies like Amazon have successfully implemented machine learning algorithms to enhance their product recommendation systems, resulting in higher customer satisfaction and increased sales.
To utilize machine learning for better results in lead generation, it is imperative to identify and improve the areas in which these models can excel. Customer segmentation, data analysis, and automation play crucial roles in this process, enabling businesses to target specific demographics, analyze data patterns, and automate repetitive tasks. By refining and fine-tuning these aspects, companies can optimize their machine learning models and maximize their lead generation efforts.
Visualizing machine learning results is another key aspect of driving better results in lead generation. Real-time insights, conversion optimization, and A/B testing can all be facilitated through effective visualization. Interactive dashboards linked to the model's REST API, such as Grafana and Prometheus, provide stakeholders with clear and actionable insights, enabling them to make informed decisions and optimize their lead generation strategies.
Explaining how machine learning models work and make predictions is essential for building trust and understanding among stakeholders. Techniques such as feature importance, partial dependence plots, SHAP values, and LIME offer insights into the inner workings of these models, allowing businesses to showcase the accuracy and reliability of their predictions. Transparency and interpretability are key when dealing with complex models or high-stakes decisions.
Evaluating the performance of machine learning models is critical in determining their effectiveness in lead generation. Metrics such as accuracy, precision, recall, F1-score, and ROC curve provide valuable insights into the model's performance on unseen data. By comparing the model's performance against a baseline model, businesses can quantify the improvement achieved and make data-driven decisions to further enhance their lead generation efforts.
Showcasing the insights generated from machine learning models is a powerful way to demonstrate their value. Key findings, actionable recommendations, and specific steps to take are all important components of showcasing the impact these models can have on lead generation. Tailoring the message and tone to the audience's expertise and interests is essential for effectively communicating the value of machine learning in lead generation strategies.
Testing assumptions and validating results are crucial steps in the refinement of machine learning models. Techniques such as cross-validation, bootstrapping, sensitivity analysis, and error analysis help identify limitations, validate assumptions, and ensure the robustness and reliability of the models. By critically evaluating the models and addressing any potential issues or challenges, businesses can improve their predictive capabilities and drive better results in lead generation.
Lastly, incorporating feedback from the audience is invaluable in refining machine learning models and improving their overall performance. By actively seeking feedback, businesses can enhance their communication skills, identify areas for improvement, and discover new opportunities or directions for their machine learning projects. Engaging with the audience fosters collaboration and ensures that the models align with the needs and expectations of the stakeholders.
Key Takeaways:
- Effectively utilizing machine learning can revolutionize lead generation and yield better results in the AI era.
- Continuous improvement and upgrading of machine learning models enhance lead generation strategies.
- Identifying and improving areas in machine learning models, such as customer segmentation and data analysis, optimize lead generation efforts.
- Visualizing machine learning results through real-time insights and A/B testing facilitates data-driven decision making.
- Explaining how machine learning models work and make predictions builds trust and transparency.
Evolution of Machine Learning Models for Improved Lead Generation
In today's AI-driven business landscape, effectively utilizing machine learning strategies can revolutionize lead generation and yield better results. Industries across the board are embracing the power of machine learning to enhance their lead generation efforts and drive growth in the AI era. By harnessing the capabilities of machine learning algorithms and predictive analytics, businesses can uncover valuable insights, optimize their processes, and ultimately attract and convert more high-quality leads.
As technology continues to evolve, machine learning models have also seen significant advancements. From simple rule-based models to sophisticated deep learning networks, the evolution of machine learning has paved the way for improved lead generation strategies. Companies like Amazon have successfully implemented machine learning algorithms to enhance their product recommendation systems, resulting in higher customer satisfaction and increased sales.
To utilize machine learning for better results in lead generation, it is imperative to identify and improve the areas in which these models can excel. Customer segmentation, data analysis, and automation play crucial roles in this process, enabling businesses to target specific demographics, analyze data patterns, and automate repetitive tasks. By refining and fine-tuning these aspects, companies can optimize their machine learning models and maximize their lead generation efforts.
Visualizing machine learning results is another key aspect of driving better results in lead generation. Real-time insights, conversion optimization, and A/B testing can all be facilitated through effective visualization. Interactive dashboards linked to the model's REST API, such as Grafana and Prometheus, provide stakeholders with clear and actionable insights, enabling them to make informed decisions and optimize their lead generation strategies.
Explaining how machine learning models work and make predictions is essential for building trust and understanding among stakeholders. Techniques such as feature importance, partial dependence plots, SHAP values, and LIME offer insights into the inner workings of these models, allowing businesses to showcase the accuracy and reliability of their predictions. Transparency and interpretability are key when dealing with complex models or high-stakes decisions.
Evaluating the performance of machine learning models is critical in determining their effectiveness in lead generation. Metrics such as accuracy, precision, recall, F1-score, and ROC curve provide valuable insights into the model's performance on unseen data. By comparing the model's performance against a baseline model, businesses can quantify the improvement achieved and make data-driven decisions to further enhance their lead generation efforts.
Showcasing the insights generated from machine learning models is a powerful way to demonstrate their value. Key findings, actionable recommendations, and specific steps to take are all important components of showcasing the impact these models can have on lead generation. Tailoring the message and tone to the audience's expertise and interests is essential for effectively communicating the value of machine learning in lead generation strategies.
Testing assumptions and validating results are crucial steps in the refinement of machine learning models. Techniques such as cross-validation, bootstrapping, sensitivity analysis, and error analysis help identify limitations, validate assumptions, and ensure the robustness and reliability of the models. By critically evaluating the models and addressing any potential issues or challenges, businesses can improve their predictive capabilities and drive better results in lead generation.
Lastly, incorporating feedback from the audience is invaluable in refining machine learning models and improving their overall performance. By actively seeking feedback, businesses can enhance their communication skills, identify areas for improvement, and discover new opportunities or directions for their machine learning projects. Engaging with the audience fosters collaboration and ensures that the models align with the needs and expectations of the stakeholders.
Key Takeaways:
- Effectively utilizing machine learning can revolutionize lead generation and yield better results in the AI era.
- Continuous improvement and upgrading of machine learning models enhance lead generation strategies.
- Identifying and improving areas in machine learning models, such as customer segmentation and data analysis, optimize lead generation efforts.
- Visualizing machine learning results through real-time insights and A/B testing facilitates data-driven decision making.
- Explaining how machine learning models work and make predictions builds trust and transparency.
Key Takeaways:
- Continuous improvement and upgrading of machine learning models can drive growth and ROI in the digital marketplace.
- Identifying areas for improvement is essential for optimizing machine learning models.
- Visualization helps in identifying patterns and making insights actionable.
- Explaining the workings and predictions of machine learning models builds trust and transparency.
Section | Summary |
---|---|
Section 2: Evolution of Machine Learning Models for Improved Lead Generation | Continuous improvement and upgrading of machine learning models is key to uncovering growth and ROI in the digital marketplace. Understanding the business problem and evaluating the model's performance are crucial steps in optimizing machine learning models for lead generation. Visualization aids in effectively displaying machine learning results, while explaining the model's workings and predictions builds trust with the audience. Key takeaways include the importance of continuous improvement, identifying areas for optimization, the role of visualization, and the significance of explaining the model's behavior. |
Identifying and Improving Areas in Machine Learning Models
To optimize machine learning models for lead generation, it is crucial to identify and improve areas that require attention and refinement. One key aspect is customer segmentation, which involves dividing the target audience into distinct groups based on similar characteristics or behaviors. By analyzing customer data and utilizing techniques like clustering or classification algorithms, we can gain valuable insights into customer preferences, interests, and purchasing patterns.
Once customer segments are identified, data analysis plays a vital role in improving machine learning models. By thoroughly examining the data, we can identify any inconsistencies, missing values, or outliers that may adversely affect the model's performance. Data cleaning techniques, such as imputation or outlier removal, can be applied to enhance the quality and reliability of the dataset.
Automation is another area that can greatly impact machine learning models. Applying automation techniques not only saves time and effort but also ensures consistent and efficient processing of data. This includes automating repetitive tasks like data preprocessing or feature engineering. By automating these processes, we can streamline the model building pipeline and allocate more time for analyzing results and improving the model's accuracy.
Benefits of Customer Segmentation, Data Analysis, and Automation in Lead Generation
Customer segmentation enables personalized marketing strategies, allowing businesses to tailor their messages, products, and services to specific customer groups. This targeted approach enhances customer engagement, satisfaction, and ultimately, improves lead generation and conversion rates.
Data analysis helps uncover hidden patterns, correlations, and trends within the data, enabling data-driven decision-making. By utilizing statistical methods, exploratory data analysis, and visualization techniques, we can gain valuable insights into customer behavior, preferences, and market trends. These insights can guide us in optimizing our machine learning models, developing effective marketing strategies, and identifying new opportunities for growth.
Automation streamlines the lead generation process by eliminating manual and time-consuming tasks, such as data preprocessing or repetitive analysis. By automating these tasks, we can ensure accuracy, consistency, and scalability in our lead generation efforts. Additionally, automation allows us to focus on higher-level tasks, such as interpreting and leveraging the insights generated from our machine learning models, leading to more effective decision-making and improved lead generation results.
Key Benefits | Customer Segmentation | Data Analysis | Automation |
---|---|---|---|
Personalized Marketing | Enables targeted marketing strategies | Reveals customer behavior and preferences | Streamlines lead generation process |
Data-Driven Decision Making | Guides effective marketing strategies | Uncovers hidden patterns and trends | Ensures accuracy and consistency |
Improved Lead Generation | Enhances customer engagement and conversion rates | Identifies new growth opportunities | Enables focus on higher-level tasks |
Visualizing Machine Learning Results for Effective Decision Making
Visualizing machine learning results through real-time dashboards and interactive visualization techniques is vital for making informed decisions and optimizing lead generation strategies. In today's fast-paced business environment, having access to accurate and up-to-date insights is crucial for staying competitive. Real-time dashboards like Grafana and Prometheus provide a dynamic and interactive way to monitor key metrics and visualize data, allowing businesses to identify trends, patterns, and anomalies that can inform strategic decision-making.
By incorporating visualizations into machine learning models, businesses can gain a deeper understanding of customer behavior, identify areas for improvement, and optimize conversion rates. Interactive visualizations enable stakeholders to explore data in real-time, drilling down into specific segments or variables to uncover valuable insights. These visualizations can also facilitate collaboration and communication among different teams, ensuring that everyone is on the same page and working towards common goals.
Tables can also play a significant role in visually representing machine learning results. They can be used to present key performance metrics, such as conversion rates, click-through rates, or customer segmentation, in a concise and easily digestible format. By organizing data in a structured manner, tables provide a clear overview of results and allow for quick comparisons and analysis.
Key Metrics | Conversion Rate | Click-through Rate | Customer Segmentation |
---|---|---|---|
Baseline Model | 10% | 15% | High, Medium, Low |
Improved Model | 15% | 20% | High, Medium, Low |
In conclusion, visualizing machine learning results through real-time dashboards, interactive visualization techniques, and well-organized tables offers businesses the opportunity to gain valuable insights, make informed decisions, and optimize lead generation strategies. By leveraging these visual tools, businesses can better understand their customers, identify areas for improvement, and drive successful outcomes.
Explaining the Workings and Predictions of Machine Learning Models
Explaining the inner workings and predictions of machine learning models is crucial for building trust and understanding their behavior, especially in complex or high-stakes scenarios. It allows stakeholders to gain insights into how the models operate, enabling them to make informed decisions based on the predictions generated.
One effective technique for explaining machine learning models is by determining the feature importance. This helps identify which input variables have the most significant impact on the model's predictions. By understanding the importance of each feature, decision-makers can focus on optimizing and enhancing the key drivers that influence the model's performance.
"Feature importance analysis can provide valuable insights into the decision-making process of machine learning models." - John Doe, Data Scientist
Another approach to understanding machine learning models is through partial dependence plots. These plots show the relationship between a specific feature and the model's predictions while holding other variables constant. By analyzing these plots, stakeholders can gain a deeper understanding of how the model's predictions change given different values of a particular feature.
In addition to feature importance and partial dependence plots, techniques such as SHAP values and LIME can be utilized to explain the reasons behind a model's predictions. SHAP values provide a unified measure of each feature's contribution to the prediction, while LIME offers local interpretability by approximating the behavior of the model in a specific instance.
Technique | Explanation |
---|---|
Feature Importance | Identify the key drivers influencing the model's predictions |
Partial Dependence Plots | Analyze how the model's predictions change with varying values of a specific feature |
SHAP Values | Quantify the contribution of each feature to a prediction in a unified manner |
LIME | Provide local interpretability by approximating the model's behavior in a specific instance |
Evaluating the Performance of Machine Learning Models
Evaluating the performance of machine learning models using appropriate metrics is essential to gauge their effectiveness in lead generation and showcasing improvement achieved. Metrics such as accuracy, precision, recall, F1-score, and the Receiver Operating Characteristic (ROC) curve provide valuable insights into how well the models are performing on unseen data.
One method for evaluating model performance is by comparing the results with a baseline model. The baseline model serves as a benchmark to measure the improvement achieved through machine learning techniques. By comparing the performance of the machine learning model with the baseline, we can showcase the value added and the enhanced predictive capabilities.
To capture a holistic view of model performance, it is important to consider multiple metrics. Accuracy measures the overall correct predictions made by the model, while precision and recall focus on the model's ability to correctly predict positive instances and identify all relevant instances, respectively. F1-score provides a balanced measure between precision and recall, offering a consolidated view of the model's performance.
The ROC curve visually displays the trade-off between true positive rate and false positive rate, allowing for the selection of an optimal threshold for decision-making. An area under the ROC curve (AUC) score helps in quantifying the model's discriminative power, with higher values indicating better performance.
By evaluating model performance using these metrics, we can identify areas for improvement and make informed decisions to optimize lead generation strategies. Continuously monitoring and assessing the performance of machine learning models ensures that they remain effective and aligned with business objectives.
Showcasing Insights Generated from Machine Learning Models
Effectively presenting the insights generated by machine learning models, tailored to the audience's expertise and expectations, is crucial for demonstrating the value they bring to lead generation. By carefully selecting and visualizing key findings, recommendations, and actions, businesses can make informed decisions and drive impactful outcomes.
To showcase these insights, one effective approach is to create interactive dashboards that provide an intuitive and user-friendly interface. Through live visualizations, stakeholders can interact with the data, explore different scenarios, and gain a deeper understanding of the underlying patterns and trends. This interactive experience allows for a more engaging and collaborative decision-making process.
Tables can be utilized to present quantitative data in a structured and organized manner. By arranging the information into rows and columns, it becomes easier for users to compare different metrics, identify patterns, and draw actionable conclusions. Additionally, incorporating interactive features such as sorting and filtering can enhance the usability of the tables, allowing users to customize the view based on their specific needs.
Key Finding | Recommendation | Action |
---|---|---|
High conversion rates for leads generated through social media campaigns | Allocate more resources to social media marketing | Collaborate with the social media team to develop targeted campaigns |
Low engagement from leads in the 18-24 age group | Modify messaging and content to resonate with younger audience | Conduct market research to better understand the preferences and needs of this age group |
Significant drop in lead quality during weekends | Adjust sales team schedules and focus efforts on weekdays | Analyze the reasons behind the drop and implement strategies to attract higher-quality leads on weekends |
Conclusion
Effectively showcasing the insights generated from machine learning models is a critical component of successful lead generation. By tailoring the presentation to the audience's expertise and expectations, utilizing interactive dashboards, and presenting data through tables, businesses can empower their decision-makers with valuable insights and drive meaningful actions for improved results.
Testing Assumptions and Validating Results in Machine Learning
Testing assumptions and validating results are vital steps in improving the robustness, reliability, and performance of machine learning models in lead generation. By assessing the accuracy, precision, recall, F1-score, and the ROC curve, we gauge the model's performance on unseen data. This evaluation provides valuable insights into the model's strengths and weaknesses, allowing us to refine and optimize its predictions.
One effective technique for testing assumptions is cross-validation. This approach involves splitting the dataset into multiple subsets, training the model on different combinations of these subsets, and evaluating its performance on the remaining data. By assessing the model's consistency across multiple folds, we can validate its ability to generalize well to new data.
Bootstrapping is another useful technique in model validation. It involves creating multiple bootstrap samples from the original dataset by randomly drawing observations with replacement. Each sample is then used to train and test the model, providing an estimate of its stability and estimating confidence intervals for the model's performance metrics.
"The most exciting phrase to hear in science, the one that heralds new discoveries, is not 'Eureka!' but 'That's funny...'" - Isaac Asimov
When encountering unexpected results or inconsistencies, sensitivity analysis can help identify potential issues and their impact on the model's performance. By varying input parameters or introducing artificial perturbations, we can explore the model's sensitivity to changes and assess its robustness.
Assessing error analysis and understanding limitations
Understanding the errors made by the model is crucial to identify limitations and improve its performance. Error analysis allows us to examine the types of mistakes the model is prone to, such as false positives or false negatives, and determine the potential causes of these errors. This analysis provides insights into areas where the model may benefit from additional fine-tuning or feature engineering to optimize its predictions.
Error Type | Potential Causes |
---|---|
False Positives | Insufficient data for certain classes or imbalanced training set |
False Negatives | Inadequate feature representation or model insensitivity to crucial patterns |
Overfitting | Model complexity, noise in the data, or lack of regularization |
By addressing these limitations and iteratively refining the model, we can enhance its accuracy, reliability, and relevance in lead generation. This rigorous testing and validation process leads to improved predictions, empowers decision-makers with reliable insights, and ultimately drives better results in utilizing machine learning for lead generation in the AI era.
Incorporating Feedback to Refine Machine Learning Models
Incorporating valuable feedback from the audience is instrumental in refining machine learning models, improving communication, and uncovering new possibilities in lead generation. As a copywriting journalist, I understand the importance of feedback in continuously enhancing the performance and effectiveness of machine learning models. Gathering feedback helps to identify areas for improvement, validate results, and ensure that the models align with the needs and expectations of the target audience.
One effective way to solicit feedback is through interactive communication channels such as surveys, user interviews, or focus groups. By actively engaging with the audience, we can understand their preferences, pain points, and expectations. This feedback not only assists in refining the algorithms but also in tailoring the messaging and tone of the lead generation strategies to resonate better with the target audience. Communication skills play a pivotal role in effectively conveying the value of machine learning models and driving engagement.
Feedback from the audience helps us fine-tune machine learning models, enhance communication skills, and discover new opportunities or directions for the project. It provides valuable insights into the needs and preferences of the audience, enabling us to iterate and improve on our approach. By incorporating feedback, we ensure that our lead generation efforts are more targeted, relevant, and effective, ultimately leading to better results.
The Power of Feedback in Lead Generation
In the evolving landscape of lead generation, feedback serves as a guiding force for refining strategies and optimizing outcomes. By actively seeking feedback from the target audience, we gain a deeper understanding of their needs, pain points, and preferences. This insight allows us to customize our machine learning models to better serve their specific requirements, resulting in more accurate lead generation and improved conversion rates.
Furthermore, feedback also plays a significant role in identifying areas for improvement within our machine learning models. By analyzing the feedback, we can pinpoint weaknesses or limitations in our algorithms and take necessary steps to address them. This iterative process of incorporating feedback ensures that our models continue to evolve and adapt to changing market dynamics, enhancing their overall performance and effectiveness in lead generation.
In conclusion, feedback is a crucial component in the success of machine learning models for lead generation. By actively seeking and incorporating feedback from the audience, we can refine our models, improve communication, and uncover new possibilities. It allows us to develop tailored strategies that resonate with the target audience, resulting in better results and higher conversion rates. As a copywriting journalist, I am committed to leveraging feedback to continuously improve and optimize lead generation efforts in the AI era.
Conclusion
In the AI era, leveraging machine learning for lead generation offers unprecedented potential for growth, ROI, and success in the digital marketplace. By continuously improving and upgrading machine learning models, businesses can enhance their lead generation strategies and achieve better results. Just like how Amazon has evolved its product recommendation system, collecting more data about products and customer behavior allows models to learn more robust and generalized patterns, reducing overfitting and improving their ability to make accurate predictions on unseen data.
To optimize machine learning models, it is important to identify areas that need improvement. Understanding the business problem and evaluating the current model's performance can help in identifying failure points and estimating the efforts required for improvement. By analyzing areas such as too many false positives or low accuracy, businesses can make informed decisions on how to enhance their models.
Visualization plays a significant role in displaying machine learning results. By visualizing data, businesses can identify patterns, correlations, outliers, and distributions, enabling better model selection and feature engineering. Real-time dashboards, such as Grafana and Prometheus, linked to the model's REST API, can provide interactive visualization of metrics, making insights clear and actionable for stakeholders.
Explaining the workings and predictions of machine learning models is crucial for gaining trust from the audience. Techniques such as feature importance, partial dependence plots, SHAP values, or LIME can be used to explain the model's behavior and provide clarity. Evaluating model performance using metrics like accuracy, precision, recall, F1-score, and ROC curve is essential for showcasing the improvements achieved. Comparing the model's performance with a baseline model further highlights the benefits of leveraging machine learning for lead generation.
To effectively communicate the value of machine learning models, it is important to showcase the insights gained. Highlighting key findings, recommendations, and actions that the models can generate helps in tailoring the message to the audience's expertise, interest, and expectations. Testing assumptions and validating results are essential steps to improve the model's robustness and reliability. By soliciting feedback from the audience, businesses can refine their models and presentations, enhance their communication skills, and discover new opportunities or directions for their machine learning projects.
FAQ
How can machine learning be utilized for better results in lead generation in the AI era?
- Machine learning can be utilized in lead generation by continuously improving and upgrading models, analyzing areas for improvement, visualizing data for better decision making, explaining model workings and predictions, evaluating model performance, showcasing insights generated from the models, testing assumptions and validating results, and incorporating feedback to refine the models.
How can machine learning models be continuously improved and upgraded for better lead generation?
- Machine learning models can be continuously improved and upgraded by collecting more data about products and customer behavior, allowing the models to learn more robust and generalized patterns. This reduces overfitting and improves the models' ability to make accurate predictions on unseen data.
How can areas for improvement in machine learning models be identified and improved?
- Areas for improvement in machine learning models can be identified by understanding the business problem and evaluating the current model's performance. Analyzing the model's failure points, such as too many false positives or low accuracy, helps estimate the efforts required for improvement.
How can visualizing machine learning results aid in decision making for lead generation?
- Visualizing machine learning results helps in identifying patterns, outliers, correlations, and distributions, enabling better model selection and feature engineering. Real-time dashboards linked to the models' REST API, such as Grafana and Prometheus, can provide interactive visualization of metrics for clear and actionable insights.
How can the workings and predictions of machine learning models be explained?
- The workings and predictions of machine learning models can be explained using techniques such as feature importance, partial dependence plots, SHAP values, or LIME. These techniques help in understanding the behavior of the models and gaining trust from the audience.
How can the performance of machine learning models be evaluated?
- The performance of machine learning models can be evaluated using metrics such as accuracy, precision, recall, F1-score, and ROC curve. Comparing the models' performance with a baseline model allows showcasing the improvement achieved.
How can insights generated from machine learning models be showcased?
- Insights generated from machine learning models can be showcased by highlighting key findings, recommendations, or actions that the models can generate. Tailoring the message and tone to the audience's expertise, interest, and expectation is crucial in effectively communicating the value of the models.
Why is testing assumptions and validating results important in machine learning?
- Testing assumptions and validating results in machine learning is important to improve the models' robustness and reliability. Techniques such as cross-validation, bootstrapping, sensitivity analysis, or error analysis can be used to test assumptions, identify limitations, or challenges faced by the models.
How can feedback be incorporated to refine machine learning models?
- Feedback from the audience helps in refining machine learning models and presentations. Inviting questions, comments, or criticisms allows for incorporating valuable perspectives and suggestions into future work, enhancing communication skills, and discovering new opportunities or directions for the projects.
New Video Course - Digital Growth Accelerator System
Check out my new digital video course that will help you to achieve ultimate digital growth based on my ‘system’
Find out more details her and sign up - https://www.amitjadhav.com/dgas/
- Amit Jadhav
www.amitjadhav.com